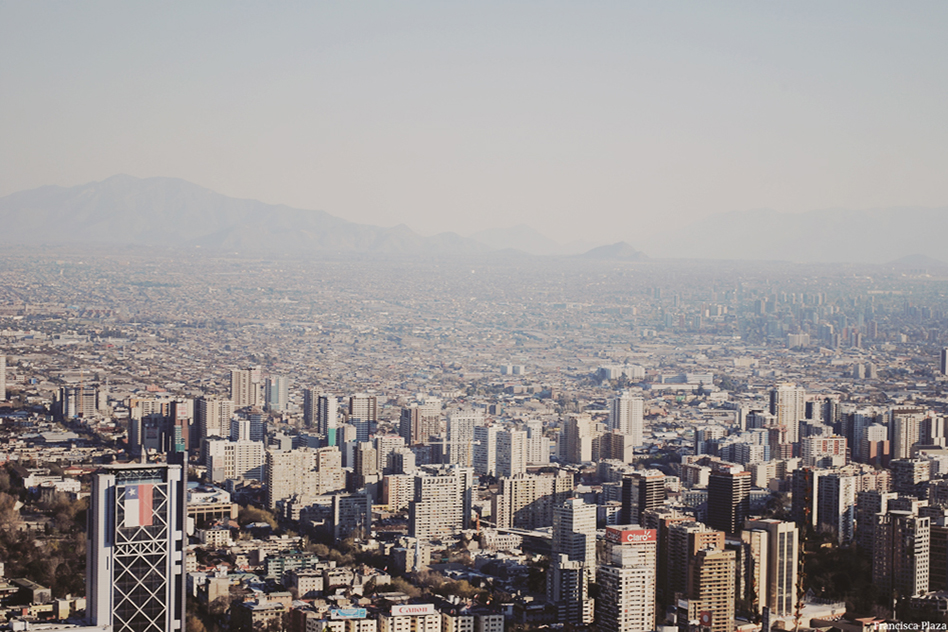
The ability to separate out a distinct signal from ambient noise in reams of scientific data is critical to detecting a meaningful trend or turning point in the data. That’s especially true when it comes to identifying signals of improving or declining air quality trends, whose magnitude can be smaller than that of underlying natural variations or cycles in chemical, meteorological and climatological conditions. This discrepancy makes it challenging to track how concentrations of surface air pollutants such as ozone change as a result of policies or other causes within a particular geographical region or timeframe.
A case in point is any attempt to estimate whether there has been any change in summertime mean ozone concentration over the Northeastern U.S., which can vary from place to place as well as from year to year. To obtain a reliable estimate in the most computationally efficient manner, one would need to know the minimum geographical area required to capture the full range of localized ozone concentrations, as well as the minimum number of years to sample to rule out short-term natural variability of atmospheric conditions—such as an abnormally hot summer or El Niño year—that may skew the numbers.
Now a team of researchers from the MIT Joint Program on the Science and Policy of Global Change and collaborating institutions has developed a method to optimize air quality signal detection capability over much of the continental U.S. by applying a strategic combination of spatial and temporal averaging scales. Presented in a study in the journal Atmospheric Chemistry and Physics, the method could improve researchers’ and policymakers’ understanding of air quality trends and their ability to evaluate the efficacy of existing and proposed emissions-reduction policies.
“Our objective was to come up with strategies, recommendations and tools to enable researchers and policymakers to determine what time scale and region to sample in order to detect a signal of a particular magnitude,” says Ben Brown-Steiner, the paper’s lead author and a former postdoctoral associate with the MIT Joint Program on the Science and Policy of Global Change and the MIT Department of Earth, Atmospheric and Planetary Sciences. “As far as I know, our study is unique in grabbing spatial and temporal variability by the horns and putting them together to maximize signal detection capability within variable and noisy data.”
Working with simulated and observed surface ozone data (with the Community Atmosphere Model with Chemistry, CAM-chem, and with the EPA’s Clean Air Status and Trends Network, CASTNET, respectively) within the U.S. covering a 25-year period, the researchers analyzed how much the variability of the data due to meteorology depended on the spatial (kilometers) or temporal (years) scale over which the data was averaged. As they homed in on the extent of the region and timeframe needed to obtain a clear signal of air quality change within the data set, they effectively determined the risk of getting an insufficiently representative sample when averaging the data over too small a region or timeframe.
As expected, they found that averaging over a greater area and timeframe, which reduces the “noise” from natural variability, will boost signal detection accuracy. The researchers’ most salient finding was that over much of the continental U.S., they could achieve the most sensitive signal detection capability by strategically combining specific spatial and temporal averaging scales. In other words, they developed a way to systematically identify a data set’s “sweet spot”—the number of kilometers and years over which to average the data so as to detect the signal most efficiently. For the hardest-to-detect signals, they recommended averaging the data over 10-15 years and over an area extending up to several hundred kilometers.
“Our results suggest that by looking at regional and/or long-term averages, we can improve our estimates of ozone levels,” says Noelle Selin, a Joint Program-affiliated associate professor in MIT’s Institute for Data, Systems and Society (IDSS) and Department of Earth, Atmospheric and Planetary Sciences, and a co-author of the study. “The results could help policymakers and others better identify the drivers of ozone pollution, and draw more robust conclusions about the impact of emissions reduction policies.”
The researchers noted that the signal detection strategies highlighted in the study may be applied not only to surface ozone data but also to a wide range of chemical and climate modeled or observational data.
The study was funded by the U.S. Department of Energy.
Photo: Smog in Santiago, Chile (Source: FRΛNCISCΛ ♦)