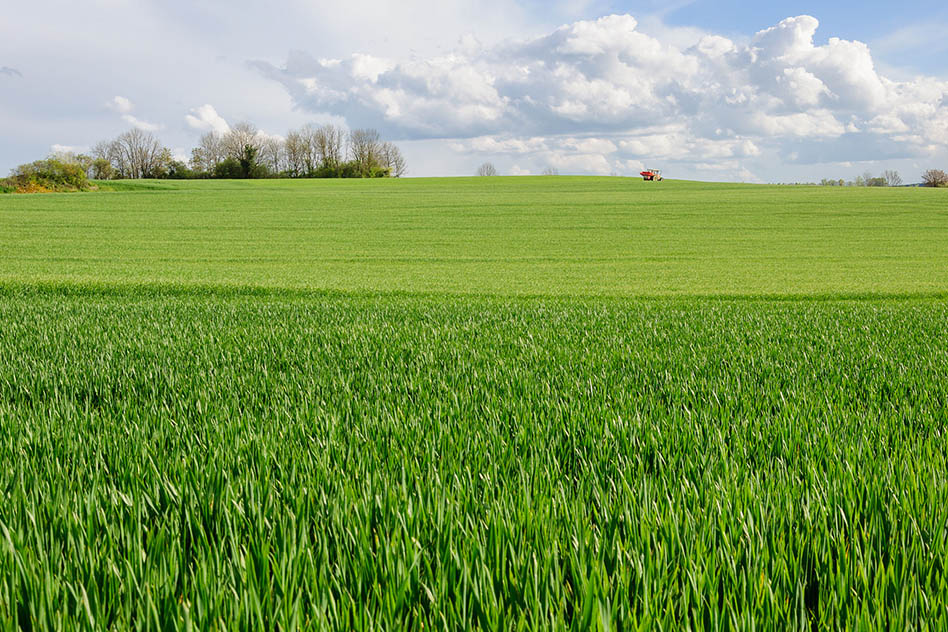
To estimate the long-term impact of climate change on crop yields, scientists usually use one of two methods. The first, process-based crop models, simulate the combined mechanistic effects of weather, soil conditions, pest damage and other factors on crop growth and yields. The second, statistical techniques, make observation-based estimates to simulate the effect of weather on crop yields. Both approaches have drawbacks.
Process-based models can simulate a wide range of weather and environmental conditions, but are computationally demanding. Statistical techniques are much more efficient, but are hampered by incomplete data sets and are ill-equipped to estimate the impacts of unprecedented climate change scenarios. Previous studies have combined the best of both methods, “training” a statistical model to make reasonably accurate predictions based on the output of a process-based model, but predictions from more than one process-based model must be considered to account for uncertainty in the impact of climate change on crop yields.
To that end, Elodie Blanc, a research scientist at the MIT Program for the Science and Policy of Global Change, has trained five simple statistical models to accurately replicate the outcomes of five process-based, globally gridded crop models under diverse climate conditions. Using the statistical models to predict the responses of maize, rice, soybean and wheat yields to climate change-driven variations in temperature and precipitation, Blanc found good agreement between predictions from the process and statistical models.
The research, which appears in Agricultural and Forest Meteorology, draws upon a previous collaboration in 2015 with Benjamin Sultan of the University Pierre and Marie Curie in Paris.
“When it comes to assessing the long-term impact of climate change on crop yields, the projected impacts of different process-based, globally gridded crop models vary widely, so it is important to consider predictions from multiple models to account for crop yield modeling uncertainty,” says Blanc. “This study’s simple, statistically-based toolset to emulate crop yields from five such models provides a reliable, more computationally efficient alternative to process-based crop models.”
Suitable for climate change impact assessments and related studies, the emulators provide a fast, easy way for researchers to estimate changes in maize, rice, soybean and wheat yields under user-defined climate parameters.
To build the emulators, Blanc’s first step was to use an ensemble of simulations from the Inter-Sectoral Impact Model Intercomparison Project (ISIMIP) to create a dataset of annual crop yields from five crop models and corresponding monthly summer weather variables for more than 100 years at the grid-cell level across the globe. She then applied this dataset to estimate, for each crop and gridded crop model, the statistical relationship between yields, temperature, precipitation and carbon dioxide. To improve the accuracy of each crop emulator (as compared to the 2015 study), Blanc employed a formula that captures the nonlinear response of crop yields to weather (including extreme temperature and precipitation events) and the effects of soil type. Validation tests showed that these statistical emulators can produce reasonable replications of crop yield results projected by the five ISIMIP crop models.
The study was funded by the U.S. Department of Energy (Office of Science), the U.S. Environmental Protection Agency and other government, industry and foundation sponsors of the MIT Joint Program.
Photo: Wheat field in Burgundy, France (Source: Myrabella)